- Crescendo
- Posts
- Is your data saying that you have a price metric problem?
Is your data saying that you have a price metric problem?
How to pick the right price metric by observing customer behavior
Welcome back to Crescendo Insights, where we provide a bite-sized piece of monetization strategy each week.
If you’re not a subscriber yet, hit the button below to keep getting these emails delivered to your inbox.
The BLUF (Bottom Line Up Front)
You can use your existing transactional and usage data to evaluate potential price metrics
The top 3 problems with price metrics: 1) your price metric doesn’t predict value, 2) your price metric has poor segmentability, and 3) you have too many metrics!
You can use advanced tools like regression analysis to act as a tie breaker between metrics
Assessing Your Price Metric with Data
Last week we went through the theory of what makes a good price metric; this week we’re diving into the data. What signals in your data can you use to identify whether you have a price metric problem and how to fix it? Next week we’ll tackle how qualitative research can help assess price metrics, so stay tuned!
Let’s dive in.
Do you have a price metric problem?
Remember the 4 elements that make a good price metric? Good price metrics need to be feasible, communicable, segmentable, and valuable. Data analysis can help you with 2 of them: segmentable and valuable. You’ll need to smash that subscribe button above if you want to hear about the other 2!
Problem #1: Your price metric doesn’t predict value
The #1 signal that you have a price metric problem is that your price is not connected to value or willingness-to-pay. That can look like a lot of things, but my favorite measure is to compare product engagement (i.e. usage) with dollars (net dollar retention or dollars paid over time).
The company below is a marketplace, so usage of the platform is measured in dollars transacted each month (the x-axis). The y-axis is the price that the customers paid to our client each year. Do you see a correlation? I don’t…

The company’s current price metric is clearly under monetizing some customers and over monetizing others, leading to high churn and poor conversion rates.
Problem #2: Your price metric doesn’t segment your customers
Your price metric is the primary way that your price scales up or down. A good price metric will enable you to widely differentiate your price among customers, often by orders of magnitude.
Below we see an example of poor segmentability: the distribution of the company’s primary price metric is listed on the y-axis, while the customer percentile is on the x-axis (notice that it’s not to scale). What do we see?

Notice how the bottom 75% of customers have either 0 or 1 of this metric. That means that for 75% of your customers, there will be no price differentiation. Then take a look at the largest customers. The top 10% of customers have a whopping 2 of this metric, and even the top 1% of customers only have 5. That means there is a 5x potential price difference between your top and your bottom customer segments. Willingness-to-pay varies FAR more than 5x in almost all markets: in B2B, it can be as much as 1,000x, but even in our Spotify Case, we saw a 30x difference.
Problem #3: Too many metrics!
Out next case involves an API company and like many API companies, pricing has become “overgrown”. In this example, the company had 5 different price metrics, each with their own limits: reads, writes, storage, databases, and integrations. Unfortunately, more metrics does not a better price model make, and our task was to simplify to see if any of the metrics were superfluous.
Below you see a good example of a metric that isn’t “pulling its weight”. That 10 million writes fence is literally not capturing a single customer (the purple dots) by a LONG shot. Having that fence there might feel safe, but its just adding confusion to an already complicated price model.

You should also check that your price metrics do not correlate with one another. If they do, you’re adding complexity without adding effectiveness and you should simplify.
In each example below, the purple dots are customers. Both are SaaS companies, but in the 1st, the company only needs to monetize 1 of the 2 metrics.

In the second, they COULD monetize both but don’t have to. Why?
Read this next sentence very carefully: just because two metrics don’t correlate, doesn’t mean you should monetize both. Only monetize both if they are both valuable and predict willingness-to-pay in different segments!

The solution: How to compare price metrics
So you’ve validated that your price metrics predict willingness-to-pay, they segment your market appropriately, and they aren’t correlated - how do you decide between 2 or many seemingly great price metrics?1
Remember, the best price metric predicts value reliably, as in when the metric is high, there is a high likelihood that the customer’s willingness-to-pay is also high.
Below is an example in the prosumer space. Our proxy for willingness-to-pay here is “product usage”, which is what we typically use in a freemium model where we don’t have historical payment activity.
In the top chart, the metric scales nicely with usage (and therefore willingness-to-pay). As we go up in customer percentile (the x-axis), we see a commensurate increase in usage. The error bars2 get wider, but that can be explained by a smaller sample as the percentiles get smaller.

In the chart below, usage doesn’t increase as regularly. Sometimes it is flat between percentile buckets (the 3rd - 5th bucket), and overall it doesn’t align with usage as well.

Cool, but what if you want to compare a bunch of metrics?3 That’s when we can put on our “fancy statistics pants”4 and break out a regression. Below is an such an example from the B2B space.
In B2B, contracts are often heavily negotiated, which means they are a better reflection of a customer’s true willingness-to-pay. In consumer, you never really know if a customer would have paid more than you charged them, but in B2B, you almost always negotiate. You can use that to your advantage by running a regression to predict negotiated price changes to list price, based on a customer’s usage of a bunch of metrics.
Below is an example with 5 metrics. While metrics A and B are predictive of a high willingness-to-pay on average, they come with large error bars indicating that they are not universally predictive of willingness-to-pay. Metric C on the other hand is much more consistent predictor of willingness-to-pay, and is a preferrable price metric. Notably, metric E negatively predicts willingness-to-pay; you should avoid pricing to that metric if at all possible.
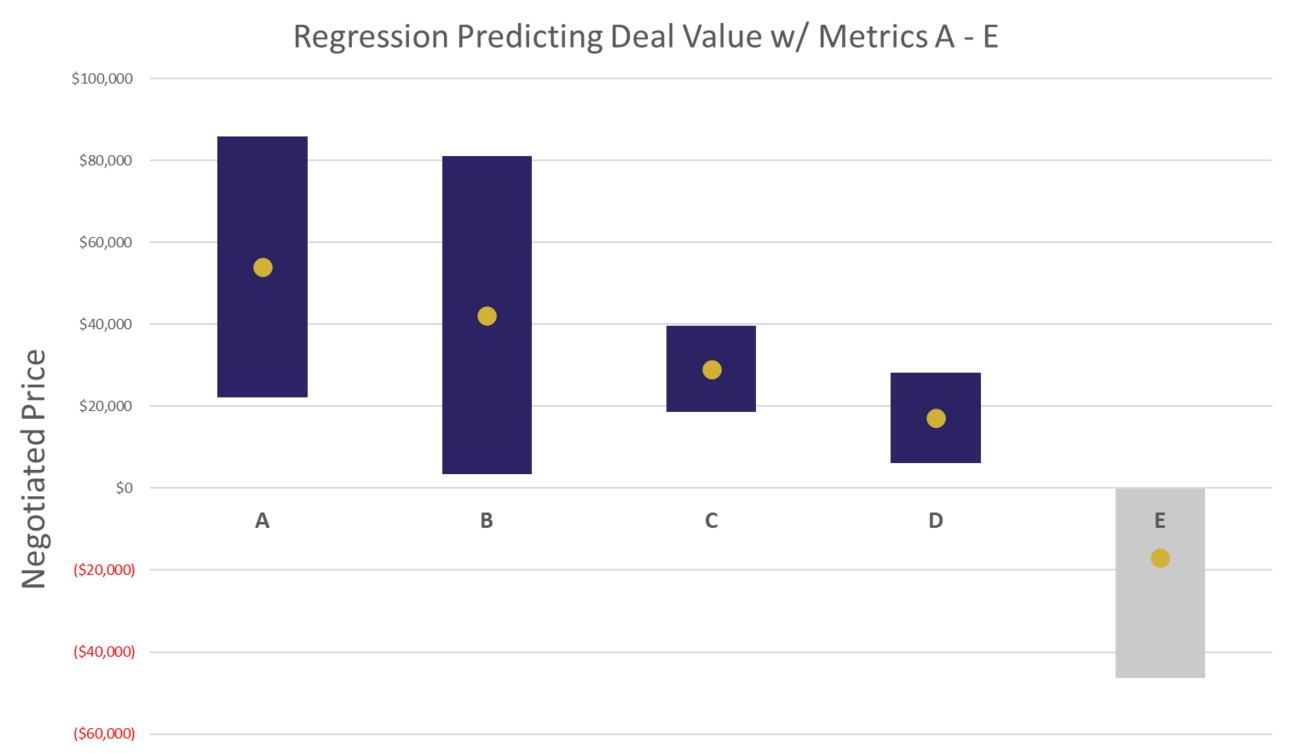
Remember, regressions are an advanced pricing tool and can be difficult to set up and interpret. I often recommend using them only after you’ve exhausted other methods of data analysis.
But what if I don’t have any data?
Data analysis is great, but it is a backward looking analysis. If you’re launching a new product or you just haven’t been keeping your data clean, these methods won’t work. You’ll have to rely on qualitative and quantitative research instead.
Tune in next week where we talk about how to assess price metric in a customer interview.
Get in touch
Crescendo works with medium-sized software companies to improve their pricing, packaging, and promotion strategies. If you’d like to book a quick consult, reach out at [email protected] or schedule time via the button below.
1 Battle Royale - pricing edition!
2 Technically the inner quartile range
3 And not overwork your poor data analysts
4 Mine are sweatpants that say “you’re significant!” on them
Reply